An improved artificial bee colony algorithm: particle bee colony
-
摘要: 針對經典人工蜂群算法收斂速率較慢,后期易陷入局部最優解的不足,本文將粒子群算法中"全局最優"的思想引入到人工蜂群算法的改進過程,從而形成了一種新的人工蜂群改進算法——粒子蜂群算法.首先,提出了趨優度的概念,用來衡量引領蜂在有限次迭代過程中向全局最優解靠近或遠離的程度,趨優度值可以評價個體的"發展潛力",趨優度值越低的個體,越需要增大變異的程度,以便找到質量更優的解.其次,專門設計了一種新的蜜蜂群體——粒子蜂,在引領蜂變異階段根據趨優度的大小將引領蜂變異為偵查蜂和粒子蜂,粒子蜂的出現在很大程度上增加了種群的多樣性,拓展了算法的搜索范圍.然后,通過粒子蜂群算法種群序列是一個有限齊次馬爾科夫鏈和種群進化單調性的分析,驗證了本文所提算法的種群序列依概率1收斂于全局最優解集.最后,將本文所提算法應用于多個常見測試函數,并與經典蜂群算法、近年其他文獻改進蜂群算法進行了仿真對比研究,仿真結果表明本文所提算法確實加大了種群的分散度、擴寬了搜索范圍,從而具有更快的收斂速度和更高的尋優精度.Abstract: With an aim to address the disadvantages of the artificial bee colony algorithm of slow convergence speed and ease of falling into the local optimum in the later period of the evolution process as well as to improve the traditional artificial bee colony algorithm, the concept of the "global optimum" in particle swarm optimization is introduced. Therefore, an improved artificial bee colony algorithm, called particle bee colony (PBC), is proposed herein. First, the concept of degree toward optimum is proposed for measuring the degree to which the leader approaches or is removed from the "global optimum" in a limited iteration process. The individuals' values of degree toward optimum denote their "development potentials." The individuals that have a low degree toward optimum require a great mutation extent to find a good solution. Second, a new colony of bees, initiated by the particle bee, is uniquely developed. In mutation period, the leader will be changed into the scout or the particle bee according to the value of the degree toward optimum. The appearance of particle bees can increase the population diversity and expand the search area to a large extent. Next, analysis reveals that the sequence of population of the PBC is a finite homogeneous Markov chain and the population evolution process is monotonous. On the basis of the above observations, it can be proved that the population sequence of the proposed algorithm converges to the global optimum solution set with probability 1. Last, the algorithm proposed in this study is applied to numerical simulations of several classical test functions. Furthermore, the proposed algorithm is compared with the traditional artificial bee colony algorithm and other improved bee colony algorithms. The simulation results show that PBC increases the population dispersion and broadens the search area, thereby allowing the proposed algorithm to achieve fast convergence rate and high optimization accuracy.
-
Key words:
- artificial bee colony /
- degree toward optimum /
- particle bee /
- Markov chain /
- population dispersion
-
參考文獻
[1] Karaboga D, Basturk B. A comparative study of artificial bee colony algorithm. Applied Soft Computing, 2008, 8(1):687 [4] Bao L, Zeng J C. Comparison and analysis of the selection mechanism in the artificial bee colony algorithm//Ninth International Conference on Hybrid Intelligent Systems. Shenyang, 2009:411 [5] Alatas B. Chaotic bee colony algorithms for global numerical optimization. Expert Syst Appl, 2010, 37(8):5682 [6] Chen S M, Sarosh A, Dong Y F. Simulated annealing based artificial bee colony algorithm for global numerical optimization. Appl Math Comput, 2012, 219(8):3575 [7] KıRan M S, GüNdüZ M. A recombination-based hybridization of particle swarm optimization and artificial bee colony algorithm for continuous optimization problems. Appl Soft Comput, 2013, 13(4):2188 [8] El-Abd M. A hybrid ABC-SPSO algorithm for continuous function optimization//IEEE Symposium on Swarm Intelligence. Paris, 2011:1 [9] Shi X H, Li Y W, Li H J, et al. An integrated algorithm based on artificial bee colony and particle swarm optimization//20106th International Conference on Natural Computation. Yantai, 2011:2586 [10] Pereira A G C, Campos V S M. Multistage non homogeneous Markov chain modeling of the non homogeneous genetic algorithm and convergence results. Commun Stat Theory Methods, 2016, 45(6):1794 [11] Sun S C, Liu H B. 6-particle swarm algorithm:convergence and applications//Swarm Intelligence and Bio-Inspired Computation, 2013:137 [12] Stutzle T, Dorigo M. A short convergence proof for a class of ant colony optimization algorithms. IEEE Trans Evol Comput, 2002, 6(4):358 -
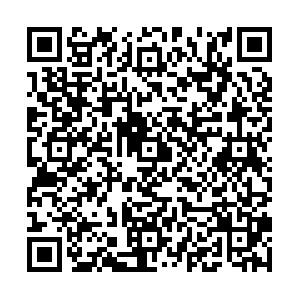
計量
- 文章訪問數: 942
- HTML全文瀏覽量: 357
- PDF下載量: 57
- 被引次數: 0