Quality prediction of the continuous casting bloom based on the extreme learning machine
-
摘要: 針對傳統基于BP神經網絡建立的連鑄坯質量預測模型訓練速度慢、適應能力弱、預測精度低等問題,本文提出一種基于極限學習機的連鑄坯質量預測方法,對方大特鋼60Si2Mn連鑄坯中心疏松和中心偏析缺陷進行預測,并與BP和遺傳算法優化BP神經網絡預測模型的預測結果進行分析對比.結果表明:BP及GA-BP神經網絡預測模型對連鑄坯中心疏松和中心偏析缺陷的預測準確率分別為50%、57.5%、70%和72.5%;而基于極限學習機的連鑄坯預測模型預測準確率更高,對連鑄坯中心疏松和中心偏析缺陷的預測準確率分別為85%和82.5%,且該模型具有極快的運算時間,僅需0.1 s.該模型可對連鑄坯質量進行迅速準確地分析,為連鑄坯質量預測的在線應用提供了一種新的方法.Abstract: To solve the problems of slow training, weak generalization ability, and low prediction accuracy in the traditional prediction model established in terms of the BP neural network, a method of the quality prediction of the continuous casting bloom based on the extreme learning machine (ELM) was proposed to predict the degree of the center porosity and the central segregation of 60Si2Mn continuous casting bloom produced by Fangda Special Steel. Comparing the prediction models of the BP neural network and the GA-BP neural network, the results show that the prediction accuracy of the model based on ELM is improved to 85% and 82.5% in the center loose and central segregation, respectively, and the operation time is reduced to 0.1 s. The model can rapidly and accurately analyze the quality of a continuous casting billet, thus providing a new method for the online application of continuous casting billet quality prediction.
-
參考文獻
[2] Bouhouche S.Contribution to Quality and Process Optimization in Continuous Casting using Mathematical Modelling[Dissertation]. Freiburg:Technische Universität Bergakademie Freiberg, 2002 [5] Huang G B, Zhu Q Y, Siew C K. Extreme learning machine:a new learning scheme of feedforward neural networks//Proceedings IEEE International Joint Conference on Neural Networks. Budapest, 2004:985 [6] Jing G L, Du W T, Guo Y Y. Studies on prediction of separation percent in electrodialysis process via BP neural networks and improved BP algorithms. Desalination, 2012, 291:78 [7] Wang Z, Chang J, Ju Q P, et al. Prediction model of end-point manganese content for BOF steelmaking process.ISIJ Int, 2012, 52(9):1585 [9] Dong S, Wang B, Wang Z, et al.Comparison of prediction models for power draw in grinding and flotation processes in a gold treatment plant. J Chem Eng Jpn, 2016, 49(2):204 [10] Hu J, Zeng X J. An efficient activation function for BP neural network//International Workshop on Intelligent Systems and Applications. Wuhan, 2009:1 [12] Liu K, Guo W Y, Shen X L, et al.Research on the forecast model of electricity power industry loan based on GA-BP neural network. Energy Procedia, 2012, 14:1918 [13] Ji C, Cai Z Z, Tao N B, et al.Molten steel breakout prediction based on genetic algorithm and BP neural network in continuous casting process//201231st Chinese Control Conference. Hefei, 2012:3402 [14] Huang G B, Zhou H M, Ding X J, et al. Extreme learning machine for regression and multiclass classification.IEEE Trans Syst Man Cybern Part B Cybern, 2012, 42(2):513 [15] Yu Q, Miche Y, Séverin E, et al.Bankruptcy prediction using extreme learning machine and financial expertise. Neurocomputing, 2014, 128:296 [16] Deo R C, Şahin M. Application of the extreme learning machine algorithm for the prediction of monthly effective drought index in eastern Australia.Atmos Res, 2015, 153:512 -
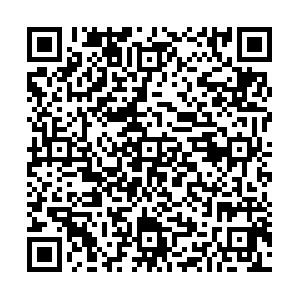
計量
- 文章訪問數: 1218
- HTML全文瀏覽量: 468
- PDF下載量: 64
- 被引次數: 0