HMM training algorithm based improved MMI and its application in facial expression recognition
-
摘要: 提出一種改進的最大互信息(MMI)準則函數并把它應用于隱馬爾可夫模型(HMM)的參數估計,重新推導了HMM的迭代公式.該準則函數相對于原來準則函數定義更為合理,能有效利用訓練樣本集中的鑒別信息,使得訓練數據得到充分利用,提高了HMM的性能.把這種改進的HMM算法應用于面部表情識別,利用改進的光流算法提取面部表情特征向量序列,并利用改進HMM算法和BP神經網絡構建了面部表情混合分類器.實驗結果表明了該方法能有效提高面部表情識別率,有效解決HMM參數估計問題.Abstract: A new approach for hidden Markov model (HMM) training based on an improved maximum mutual information (MMI) criterion was presented and HMM parameter adjustment rules were induced. By adopting a more realistic MMI definition, discriminative information contained in the training data could be used to improve the performance of HMM and this method was also used in facial expression recognition. Facial expression feature vector flows were extracted by using the improved optical flow algorithm, and a hybrid classifier based on the improved HMM and BP neural network was designed. Experimental results show that the new method provides satisfactory recognition performance and the method is powerful for HMM parameter estimation.
-
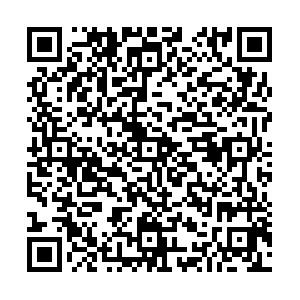
計量
- 文章訪問數: 163
- HTML全文瀏覽量: 15
- PDF下載量: 4
- 被引次數: 0