Neural networks hardware implementation based on FPGA
-
摘要: 提出了一種可以靈活適應不同的工程應用中神經網絡在規模、拓撲結構、傳遞函數和學習算法上的變化,并能及時根據市場需求快速建立原型的神經網絡硬件可重構實現方法.對神經網絡的可重構特征進行了分析,提出了三種主要的可重構單元;研究了可重構的脈動體系結構及BP網絡到該結構映射算法;探討了具體實現的相關問題.結果表明,這種方法不僅靈活性強,其實現的硬件也有較高的性價比,使用一片FPGA中的22個乘法器工作于100MHz時,學習速度可達432MCUPS.Abstract: For different engineering applications, neural networks varied in scale, topology, transfer functions and learning algorithms. A reconfigurable approach for neural hardware implementation was proposed, which was not only flexible to meet those changes, also with the fast prototyping ability for market requirements. Three kinds of reconfigurable processing units were presented based on the analysis of neural network's reeonfiguration. A reconfigurable systolic architecture was put forward and the method of mapping BP networks into this architecture was introduced. Implementation issues were discussed with an example. The results showed that a high learning speed of 432 M CUPS(Connections Updated Per Second)was achieved (working at 100 MHz using 22 multipliers) at a reasonable cost.
-
Key words:
- neural networks /
- FPGA /
- reconfigurable /
- systolic
-
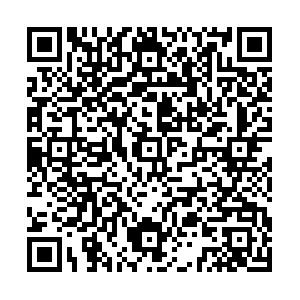
計量
- 文章訪問數: 152
- HTML全文瀏覽量: 25
- PDF下載量: 9
- 被引次數: 0