Fault diagnosis system based on ICA feature
-
摘要: 針對礦山破碎機的聲音故障診斷受復雜現場環境制約、確診率低的難題,結合獨立分量分析(ICA)在自然圖像和連續語音信號中特征提取的方法,采用兩層ICA分別用于從混雜聲音中提取各采集通道(部位)的統計獨立聲音信號和進一步提取該信號的特征基.訓練階段生成的特征基系數序列用來生成矢量量化(VQ)的碼書,設計出ICA-VQ破碎機故障診斷系統.現場采集數據的實驗中系統的故障診斷準確率達到96.8%,表明系統的高效性.Abstract: To overcome the difficulty of complex background in mining machine fault diagnosis, a fault diagnosis system based on independent component analysis (ICA) and vector quantization (VQ) was developed. A fault sound ICA model was presented to get the fault sound feature bases with ICA algorithms in extracting nature images and continuous speech features. One ICA separated the sounds from different parts of the machine and the other extracted the feature basis of fault sound. The coefficients of the basis were used in designing codebooks. The diagnosis accuracy of this system is 96.8% in the experiment with the realistic mine machine fault data, so the ICA-VQ is a high efficient fault diagnosis system.
-
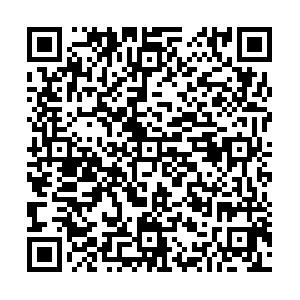
計量
- 文章訪問數: 164
- HTML全文瀏覽量: 22
- PDF下載量: 7
- 被引次數: 0