Nonlinear time series noise reduction method based on phase reconstruction and principal manifold learning
-
摘要: 提出了一種基于相重構和主流形識別的非線性時間序列降噪方法.帶噪的時間序列在高維的相空間中其本質特征隱含在一個低維的主流形中,利用局部切空間變換方法提取其主流形,再根據主流形對時間序列進行重構,就可以達到降噪的目的.與現有的非線性時間序列消噪算法不同,基于主流形的消噪算法更強調時間序列的整體結構.數值仿真分析的結果驗證了該降噪方法能有效地消除非線性時間序列中的高斯白噪聲.Abstract: A noise reduction method in nonlinear time series based on phase reconstruction and manifold learning was proposed. In a high dimensional phase space, the inherent features of time series were exhibited as a low dimensional nonlinear principal manifold. The noise was reduced by the reconstruction with the underlying manifold which was obtained through a local tangent space alignment algorithm. Different from the existent noise reduction methods in nonlinear time series, the method based on principal manifold learning emphasized more on the global structure of time series. The results of numerical simulation proved that the method could remove the Gaussian white noise in nonlinear time series effectively.
-
Key words:
- noise reduction /
- manifold learning /
- phase reconstruction /
- nonlinear time series
-
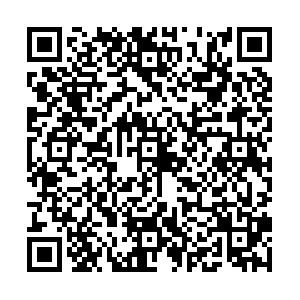
計量
- 文章訪問數: 143
- HTML全文瀏覽量: 17
- PDF下載量: 6
- 被引次數: 0